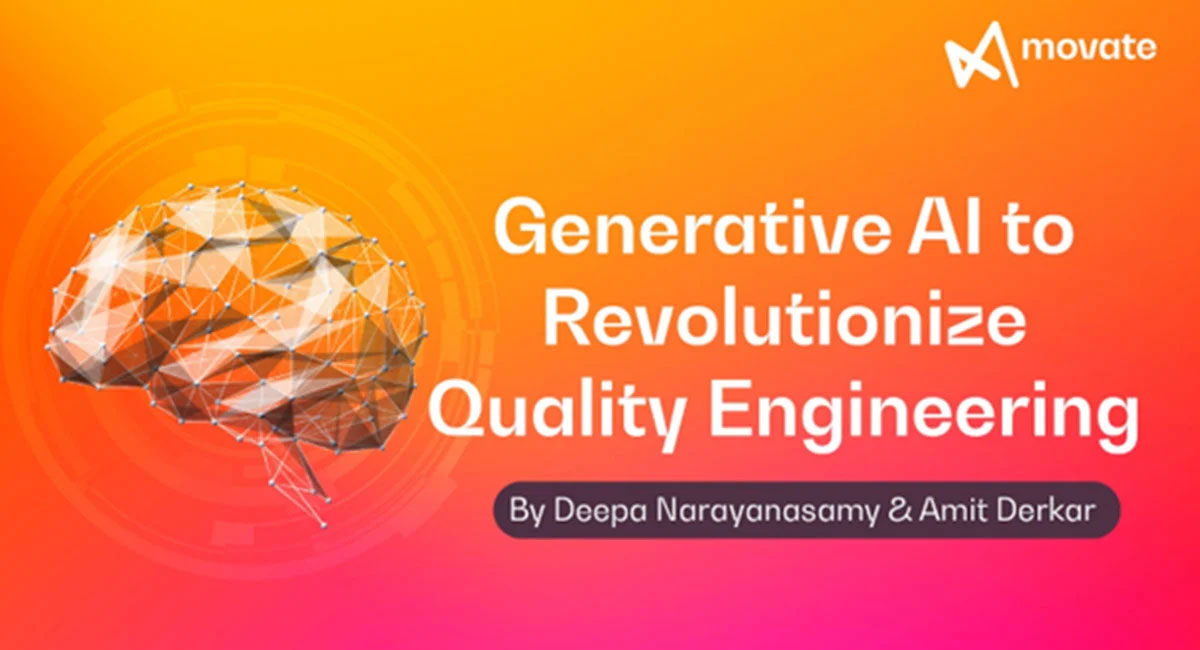
By Deepa Narayanasamy & Amit Derkar
In today’s era, delivering consistent and superior quality of experience across channels with speed & scale is a critical competitive differentiator for global enterprises. As a result, Quality assurance/Testing, a pillar of the software development lifecycle, is undergoing a tectonic shift. As of 2023, organizations engaged in software development are investing 31% of their total budget into QA & testing. Organizations are investing in leveraging Generative AI to improve its accuracy, efficiency, effectiveness, and cost-efficiency. Every alternative article or post in LinkedIn revolves around Generative AI, and everyone is trying to look at how this disruptive technology is going to impact the way we live and work. Explore how GenAI to to revolutionize QE (Quality Engineering), enhancing efficiency and performance across industries. Generative AI has the potential to significantly enhance the efficiency and effectiveness of the Quality Engineering (QE) process. By automating certain tasks and providing insights & recommendations based on data analysis, generative AI improves the quality of software systems and reduces the time & resources required to test & validate them. It can lead to more reliable software, faster delivery times, and a more simplified & efficient QA process.
Gartner predicts by 2025, 30% of enterprises will have implemented an AI-augmented development & testing strategy, up from 5% in 2021.
The Power of Gen AI for New Age QE
With the shift from the traditional Quality Assurance (QA) to the new age Quality Engineering (QE) approach, the spotlight is on automation [in fact, automating the existing automation!] The role of testing becomes increasingly critical when organizations strive for high quality deliverables with tight timelines. Utilizing Generative AI for testing helps in empowering testers & enhancing software quality. Generative AI’s powerful capabilities hold much promise in tackling the challenges of traditional testing methodologies. It’s ability to solve high complex problems ensures zero slippages via comprehensive testing and coverage. Generative AI understands requirements and generates test cases that we can subsequently expand and enhance. With training, it can be enhanced based on past defects, test cases to improve test generation and execution quality, resulting in faster testing cycles, enhanced accuracy, and higher software quality. We can draw upon the benefits from innovative use cases to transform the current enterprise testing ecosystem across the entire lifecycle and empower the shift-left approach.Gen AI helps programmers & software developers in certain laborious tasks such as code optimization, bug detection, and code completion. – AI Multiple.
Five Compelling Use Cases
Here are some scenarios worth examining. These cases are where we see the maximum benefits of Generative AI in testing to potentially occur.- Automated test case generation: Will AI generate accurate test cases? May not be, but we can enhance its outputs. Let’s take the example of a swagger API, where you can input a URL and expect test case outputs. We may not be able to utilize the results as is, but we can build upon it. We, as humans, lack test coverage of all the possible combinations of scenarios and we can use AI’s helping hand to close the gap. A trained model assisted with multiple sources of inputs like user acceptance criteria, existing test cases, technology & product domain information, may be able to analyze the requirements holistically and produce test scenarios & test cases covering almost all combinations of inputs, outputs, and even the edge cases. Integrating Gen AI in testing can significantly accelerate testing and delivery.
- Synthetic test data generation: Why not leverage AI techniques to generate realistic, synthetic test data for testing? It helps to cover an extensive number of scenarios including edge cases, large volume of data generation in a short time frame, complex data that mirrors real time scenarios, mimicked & mocked data that ensures security, etc. This lets us focus more on other critical aspects of testing and leaving AI to focus on data preparation. We only spend time reviewing and implementing.
- Gen AI for NFRs: We always think about functional testing, speed, quality, why not some performance testing, usability testing and some compliance testing? We can look at AI to identify and generate some possible performance & other non-functional use cases. Leverage the same for better outcome as we do for a functional case. By creating large volumes of data and emulating different user patterns, GANs help in assessing the application’s performance under different workloads. This is something we can explore, learn, and implement.
- Realistic user behavior simulation: Generative AI could play a crucial role in simulating realistic user behavior during application/UI testing, enabling comprehensive assessment of user experience. Again, having been trained on real user interactions, it could model & simulate realistic user behavior, to identify potential usability issues, and deliver a more seamless user experience.
- Risk-based testing: Gen AI can assist in prioritizing testing efforts based on risk analysis. It can learn from past test execution, defect patterns and performance. With this, AI can recommend test scenarios with the highest potential for uncovering critical defects. This way, we can focus on most impacted application areas thereby improving efficiency.
Gen AI will hasten the pace of modern software development, foster experimentation and transform the present software engineering funnel in the future. – Forbes
- However, while generative AI is undoubtedly a powerful tool, it is important to approach generative AI with caution as the technology is evolving. We must recognize its limitations as well as its potential benefits.There are challenges in using Gen AI in terms of accuracy, interpretability and it can be biased. Here are some of the challenges in using generative AI for testing:
- Accuracy: Generative AI models can be inaccurate or even incorrect. Human supervision of the output is still required to ensure tests, data, are correct and in a testable shape.
- Bias: Generative AI models can be biased, which can lead to tests that are not representative of the real world. Tests or data that is generated tends to mirror the input data and sources. Hence, the quality of input is key to the quality of output.
- Interpretability: Generative AI models can be difficult to interpret, which can make it difficult to understand why a test case was generated or why a test failed.
Shift, Pivot, & Adapt
Gen AI promises to revolutionize QE to bring in efficiency, accuracy, and comprehensive coverage. From enabling the creation of high-quality software to facilitating faster deliveries & achieving comprehensive test coverage, by automating test case creation, enhancing bug detection, and simulating realistic user behavior, Generative AI will transform traditional testing methodologies and is likely to propel software development to new heights. As this technology continues to evolve, its potential for further advancements in quality assurance, efficiency, and overall software excellence is exciting.
Identifying the Right Use Cases
Organizations need to identify the right use cases of Generative AI in the QE domain. These use cases can bring true competitive advantage and can create the largest impact relative to existing solutions. Once the right use case is identified, they will need to work with their technology teams responsible for QE & Testing ecosystem to define the feasibility and roadmap of leveraging Generative AI capabilities in QE.Partner with Movate
With Movate’s 18+ years of experience in delivering memorable customer experience and our dedicated focus and investments are towards building Generative AI capabilities and services for our clients. Movate helps enterprises to set up a Generative AI Co-innovation Lab to accelerate the adoption of Generative AI across the enterprise. The journey towards fully leveraging Generative AI in QE is complex and filled with learning curves, but the destination promises a bright future. As Generative AI continues to evolve and mature, Movate would like to partner with Global enterprise and their QA teams to help them deliver high-quality, reliable, and robust software.
- Contact us for help in ideation, consulting, use-case development, co-engineering and delivery.
Additional Information
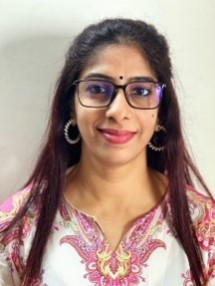
Deepa Narayanasamy is the Technical Test Architect for Digital Engineering Services (DES) at Movate. A seasoned professional with 16 years of experience in the testing industry. She has played diverse roles such as Test Program Lead, Automation Architect and independently led the successful delivery of complex automation programs. Deepa has effectively collaborated with a wide range of customers across various domains, from conducting PoCs and PoVs to devising automation strategies, scaling teams, and supporting delivery with technical leadership.
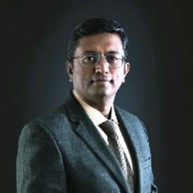
Amit Derkar is a go-to-market professional with 15+ years of experience in offerings development, sales enablement, proactive sales, analyst relations and strategic research across digital areas: marketing, commerce, data & analytics, cloud and IoT. He has been instrumental in shaping proactive sales propositions in collaboration with sales, presales and CoEs/Practices. Amit is part of the core M&A team to assess the right target companies for strategic acquisitions.